NHF Finance Review: Artificial Intelligence (AI) in Housing Finance – Is This The Future?
At the NHF Finance Conference held in March 2024 at the ACC in Liverpool, Mobysoft and Together Housing, alongside Bromford, discussed how AI is impacting finance teams across social housing providers in the UK. There were a lot of questions and interactions from the audience, so we thought we would summarise some of those along with the responses. If you would like to find out more about AI in social housing then please do download our Introducing AI Into Your Organisation guide.
To what extent do you expect LLMs (Large Language Models) to be leveraged over the medium term?
Large Language Models (LLMs) are generalised AI (i.e. not domain specific), as they are not trained on housing data but on huge general datasets. But, of course LLMs can help. For example, landlords can integrate ChatGPT and other LLMs into applications such as customer service bots. There are a plethora of simple scenarios how LLMs can help including: tenant communication and support, automated document processing, customer feedback analysis, and language translation etc.
However, there is an important point that landlords need to address first. If they going to build and maintain these applications, it’s important to consider that AI is unlike other software – it needs monitoring and continual assessment. If landlords have dedicated ongoing resource then this can be managed, if not however, it may be prudent to invest in third party applications from dedicated providers.
The above addresses the short term in reality, predicting the medium term is much more difficult with the rate of change that AI is consistently delivering.
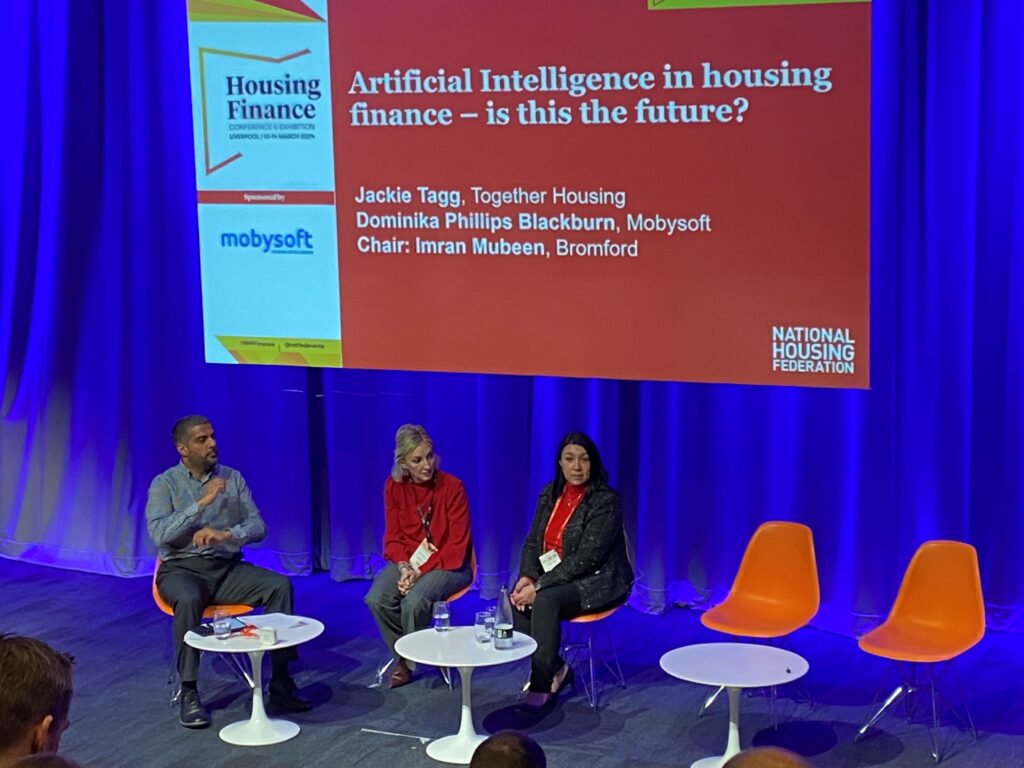
Does it work for smaller datasets? Is intelligence obtained across several organisations?
AI has been developed on large datasets (as the term Large Language Models implies.) Getting AI to effectively to work across small datasets is difficult and it could limit its effectiveness. The larger the dataset and the better quality the data, the better the output.
How does AI support an understanding of repairs trends? And how has it been linked to strategic asset management plans?
There are some key areas that AI can help in understanding repairs trends. By using data analysis and pattern recognition it can analyse historical repair data to identify patterns and trends, whether that be geographical, component focused, property type, weather, and so on.
The caveat to all this is the size of the and quality of the dataset. For purposes of context, Mobysoft’s RepairSense repairs platform has analysed over 7 million repairs jobs, and that number is growing all the time.
Natural Language Processing (NLP) is an AI technique that can be used to analyse unstructured data sources (such as repairs notes, tenant comments and so forth). AI can identify recurring issues, assess tenant satisfaction levels, and prioritise areas for improvement.
Machine Learning Models can be trained on historical repair data to identify factors influencing repair trends and predict future outcomes, such as anticipate demand fluctuations, and support data-driven decision-making in repair planning and resource allocation.
With better data insight and more data-driven decision-making, this can be used for longer term strategic asset management.
How can AI help social landlords deliver VfM? You mentioned asset management and repairs, can it give us lessons learnt information to help aid future decision making?
If AI is used to improve repair services for example, by predicting maintenance, prioritising repairs and the like, this in turn impacts customer satisfaction but also reduces spend on ongoing repairs and maintenance (which in turn helps deliver better VfM).
One of the key drivers of AI is to support data-driven decision-making in repair planning and resource allocation, which is learning lessons from historical data that in turn helps aid future decision making.
What are the drivers of arrears and which interventions have been most effective in tackling arrears?
There are many drivers of arrears, as everyone’s situation is unique, but the environmental pressures at the moment are arguably the most challenging they have been for tenants in many years. Speaking to practitioners, it’s apparent that the best interventions are early and supportive, as this helps prevent arrears from increasing. Indeed, the most effective method is prevention – where organisations are able to prevent people from falling into arrears in the first place.
Where do the panel see changes in technology and AI taking us in the next 5 -10 years?
Predicting even two years ahead with AI is difficult enough due to the rapid pace of change, but predicting what the next 5-10 years will look like is nigh on impossible.
A common belief is that AI will become infused in most software in the next two years, and help deliver huge efficiencies. From design, accounting, administration, customer services, repairs and so on. Tools like Microsoft Co-Pilot will reduce personal administration hugely, managing diaries, summarising meetings and actions and other repetitive tasks.
The biggest challenge will be cultural – embracing this change and utilising the technology effectively. It’s for this reason that organisations with AI councils and AI champions will be more successful than those with a reluctant culture.
For further exploration of some of the key considerations when adopting AI, check out Mobysoft’s Introducing AI Into Your Organisation guide. Click the image below to download your copy today!