Artificial Intelligence: A Solution to Damp and Mould Issues for Social Landlords?
The social housing sector has been under scrutiny recently resulting from multiple incidences of damp and mould issues adversely affecting the lives of tenants across the UK. Perhaps unfairly however, the resulting media coverage has painted a picture of uncaring landlords putting profits over people. But this simple narrative doesn’t delve deep enough into the underlying causes of the problem and is extremely reductive in its framing of the situation…
Is Unstructured Data Enabling Inaction?
In order to effectively and efficiently identify potential damp and mould issues affecting tenancies, landlords often have to rely on analysis of unstructured data (i.e data that is not defined or arranged according to set criteria) and it’s this from which the problems arise.
For social landlords to get a clear view of all their properties and understand which have been affected by damp and mould they are often required to interpret data sets from multiple systems, such as housing, asset or risk management systems, mobile workforce systems and contractors’ own systems. These systems are generally not integrated, meaning a further headache arising from different personnel having different levels of access across an organisation, or in the case of third-party contractor systems, sometimes no access for the housing provider at all.
Combine this with the fact that unstructured data, by its very nature, is unlabeled, emanates from disparate streams, and is devoid of any context and it’s easy to see why it is hard for social landlords to see the wider picture when it comes to assessing and understanding the damp and mould issues across all their tenancies.
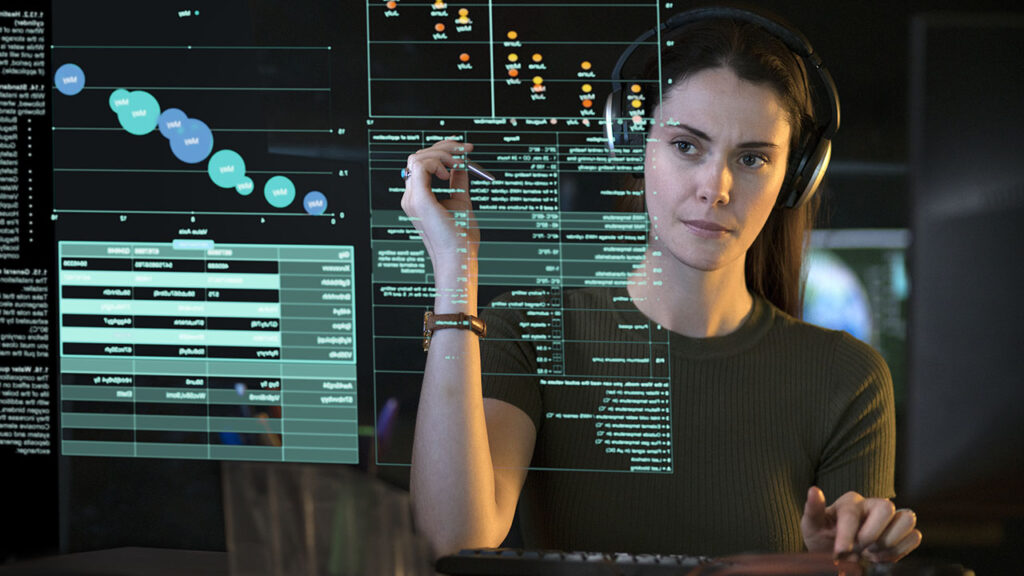
Getting Priorities Right
Identifying those properties that have been impacted by damp and mould is the first concern for social housing providers approach to the problem. The second is the ensuring that they have the ability to assess the seriousness of individual damp and mould cases so tenants can be accurately prioritised and resource allocated to resolving the situation.
Mobysoft customer success manager, Zoe La Brow, says of the current situation: “There is a perception in the media that landlords are uncaring about damp and mould across their properties. However, we know landlords are working incredibly hard to try and support and resolve these issues across their properties for their tenants.”
“Data pertaining to damp and mould, for many landlords, is recorded in unstructured data sets – the data is not defined or arranged according to set criteria and spans multiple systems across organisations, making it difficult for any user to get a full picture of the issue across the full quota of an organisations’ tenancies”
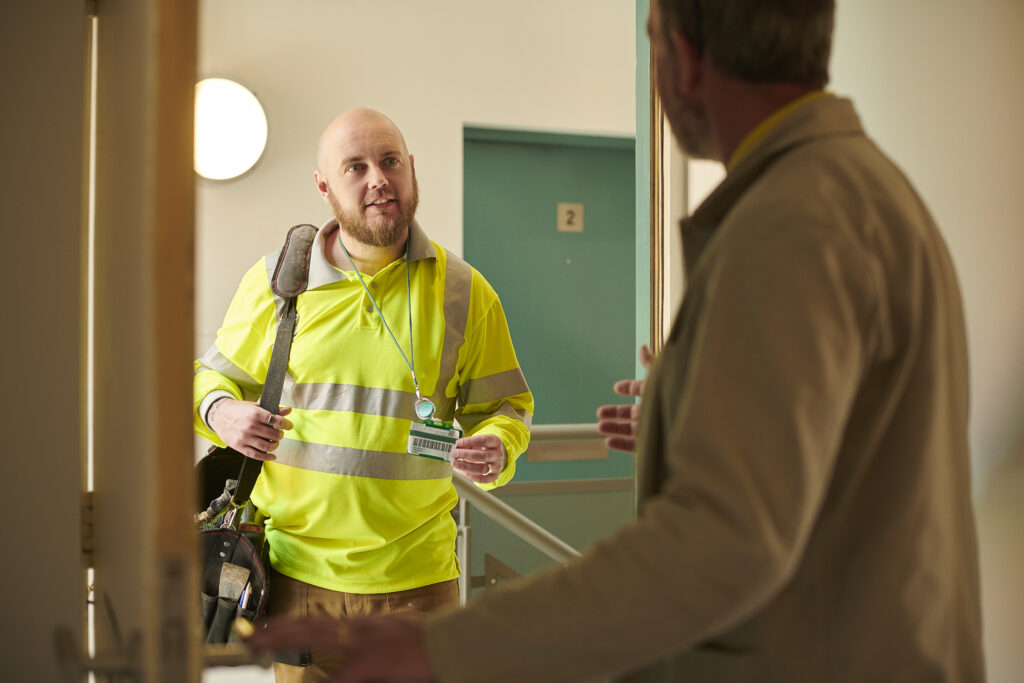
There’s No Other Way?
In order to remedy this situation in which landlords must contend with a mish-mash of tangled data, Mobysoft developed over the past three years an artificial intelligence-powered platform for repairs (RepairSense), that helps landlords improve the quality and sustainability of their repairs service. Through its use of supervised machine learning and data science, RepairSense has analysed over 2 million repairs jobs and created labelled data, the results of which allow users to surface the right data stream, analyse it, and attribute resources accordingly.
By identifying priority cases, supplying complete information about a case and actioning appropriately, front-line repairs operatives are also empowered and incentivised to focus on longevity of repair as opposed to ‘first time fix’. The results so far have seen improved productivity, reduced the number of repairs and most importantly improved customer satisfaction, the latter being especially pertinent to social landlords given the forthcoming Tenant Satisfaction Measures.
The RepairSense platform has the ability to identify and prioritise those tenancies with damp and mould issues with precision. By identifying how many times a specific issue has arisen and over what period and flagging accordingly, a clearer picture of the problem is painted. In doing so it removes the need for resource-sapping and sometimes unreliable manual analysis by landlords and enables them to focus on resolving issues for their tenants.
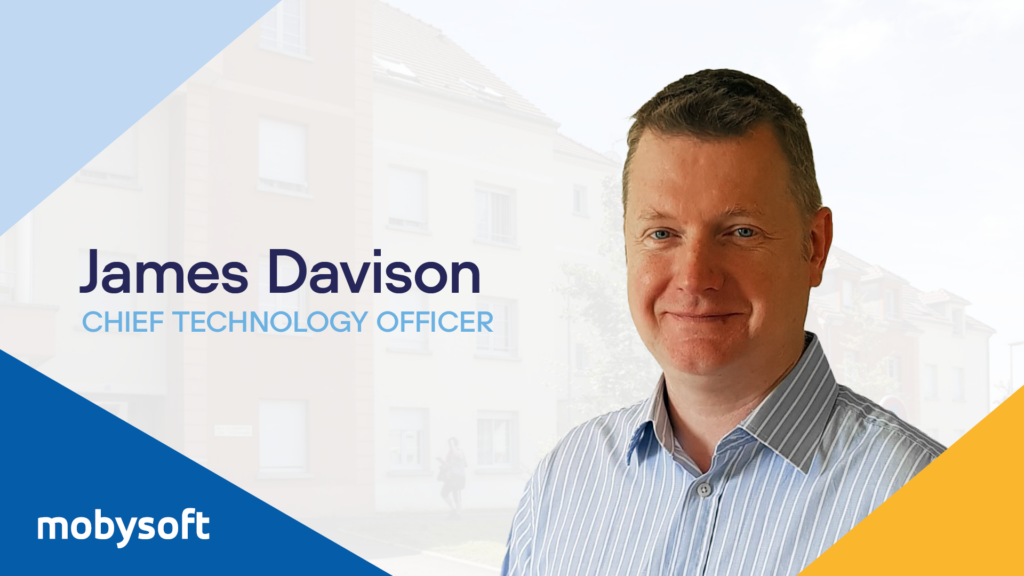
Data Is Key
Mobysoft CTO, James Davison, said: “This is just a small element of what the platform can deliver – we are developing further insights for damp and mould, specifically on our predictive analytics engine to help flag unreported damp and mould issues across all tenancies.
“Given the size of the dataset we have across the platform, unrivalled by any other data-insight providers in the sector, we are developing the most effective fix for specific repairs issues, that social landlords can depend upon to drive operational efficiencies and drive performance.”
If you’d like to learn more about how RepairSense can help your organisation’s repairs service tackle damp and mould then fill out our contact form and book an appointment with a member of our RepairSense team.
- This Month In Social Housing: September 2024 - September 30, 2024
- Customer Success Snapshot: Greatwell Homes - September 17, 2024
- This Month In Social Housing: August 2024 - August 30, 2024